In a recent milestone achievement, the Intelligent Management and Control Team within the esteemed Vehicle Engineering Department at our university has charted pioneering advancements in the domain of power battery health state estimation. Their groundbreaking research, titled "Data-Driven Transfer-Stacking based State of Health Estimation for Lithium-Ion Batteries," has been showcased in the esteemed IEEE Transactions on Industrial Electronics—an apex journal renowned for its eminence in the realm of automation and control systems. Teacher Wu Ji of our institution leads as the first author, with Lin Mingqiang, an associate researcher from the Fujian Institute of Material Structure, Chinese Academy of Sciences, assuming the role of corresponding author. Collaborating on this monumental endeavor are Mr. Meng Jinhao, associate researcher of Xi'an Jiaotong University, and Mr. Peng Jichang from Nanjing Institute of Technology, serving as co-authors. This prolific research venture received substantial support from the National Natural Science Foundation of China, the Pilot project of Young Science and Technology Talents Support Program for Anhui Association for Science and Technology, and the Program B project of Hefei University of Technology.
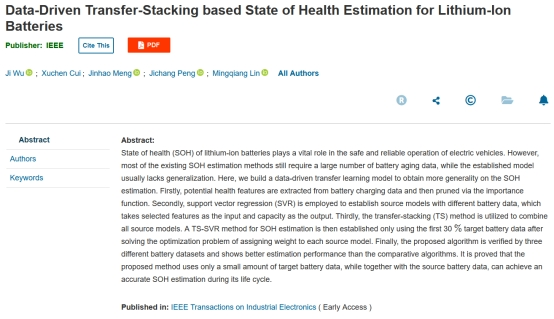
The crux of this groundbreaking exploration lies in the pivotal role of the lithium-ion battery's health state, commonly known as the State of Health (SOH), in accurately gauging the batteries' aging status—a critical facet ensuring the safe and dependable operation of electric vehicles. Nonetheless, two primary challenges have pervaded the study of SOH estimation methods: firstly, the requirement for a voluminous dataset of battery aging data to construct precise models, which poses a hurdle when only limited data is available; secondly, the existing research utilizing machine learning methods often lacks the requisite generalization ability of data-driven models. Many studies tend to establish specialized aging models for individual batteries, neglecting the disparities between batteries. To address these challenges, the team embarked on a concerted effort to amalgamate the Support Vector Regression method (SVR) and the Migration Stacking method (TS) to devise an SOH estimation method boasting robust generalization capabilities.
Initially, the team extracted essential health-related features from battery aging data, employing the decision tree's node impurity function to sieve and retain characteristics exhibiting strong correlations with battery aging. Subsequently, the team deployed the Support Vector Regression and Migration Stack methods to model multiple batteries, optimizing weights in stacked models through weight optimization functions, culminating in the development of the final migration stack model. Rigorous validation across three sets of battery data affirmed the efficacy of the team's approach, demonstrating precise SOH estimation even with limited data. Notably, the team showcased the SOH estimation curve and error distribution from NASA random condition cells, wherein their proposed TS-SVR method outperformed other methods based on experimental results.
In recent years, harnessing the intellectual might of the Anhui Province Traffic Road Collaborative Engineering Research Center, the New Energy Power System Intelligent Management and Control Team have fervently addressed scientific and technical challenges within the domains of vehicle and new energy sectors. Their extensive research, encompassing battery system modeling, simulation, state estimation, and optimization management, has yielded a series of impactful research outcomes, contributing significantly to the fortification and development of vehicle engineering and new energy science and engineering majors.
(Research Paper Link: https://doi.org/10.1109/TIE.2023.3247735)
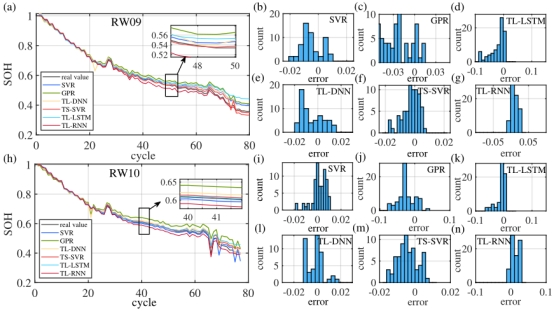